Embracing change and resetting expectations
Professor of Mathematics at University of California, Los Angeles
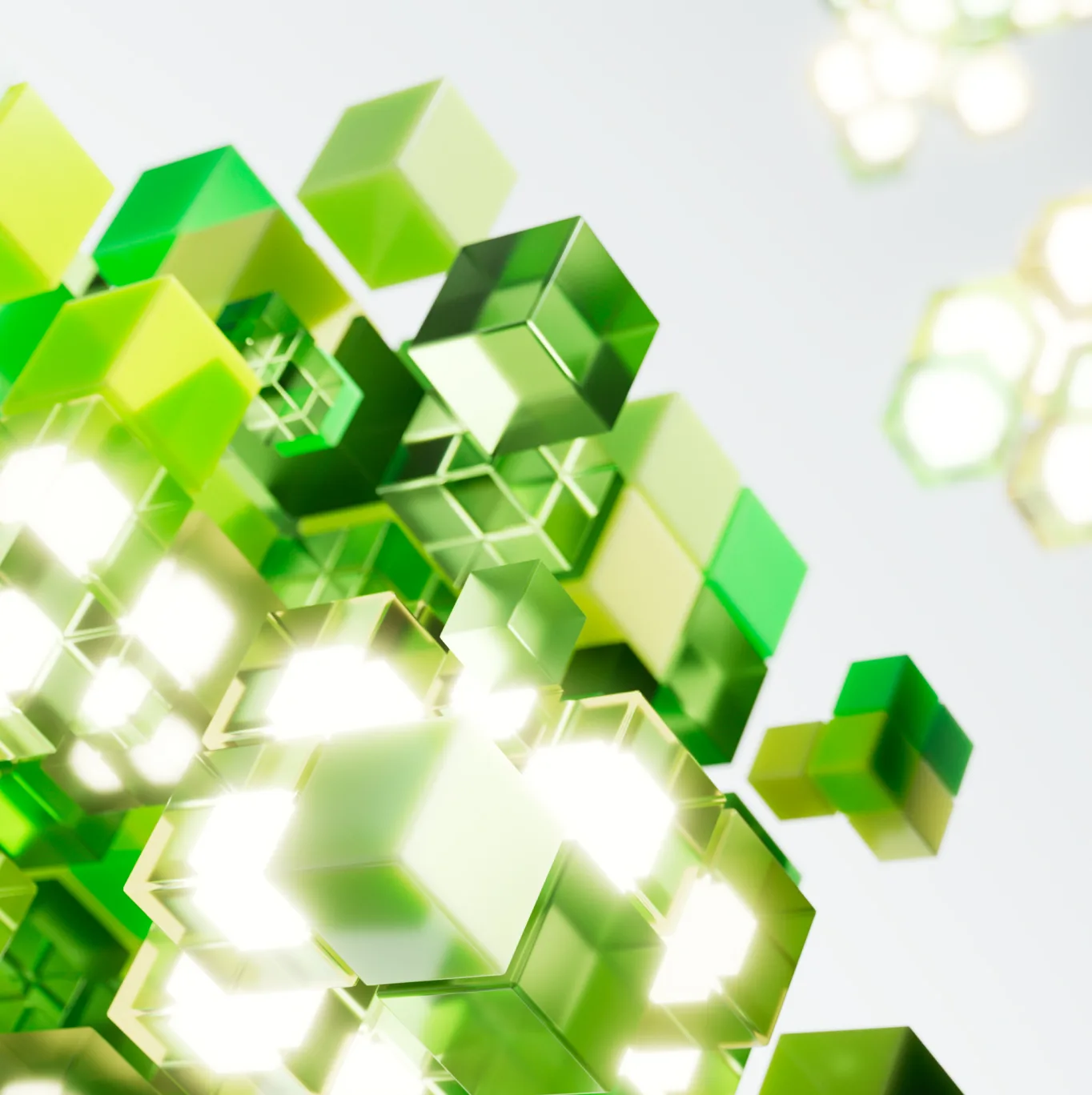
Humans have been trained over the last few decades to expect certain things from information technology. To list a few:
- Hardware and software will improve (in such metrics as performance, user experience, and reliability) at a Moore’s-law type of pace, before transitioning to more incremental improvement.
- Individual software tools can reliably produce high-quality outputs, but the input data must be of the highest quality, carefully formatted in the specific way that the tool demands.
- The more advanced the tool, the more complex the specifications and edge cases, making interoperability between tools (particularly between different providers) a significant technical challenge unless well-designed standards are in place.
- The humans will make all the key executive decisions; the software tool influences the decision-making process through its success or failure in executing human-directed orders.
All of these expectations will need to be recalibrated, if not abandoned entirely, with the advent of generative AI tools such as GPT-4. These tools perform extremely well with vaguely phrased (and slightly erroneous) natural language prompts, or with noisy data scraped from a web page or PDF. I could feed GPT-4 the first few PDF pages of a recent math preprint and get it to generate a half-dozen intelligent questions that an expert attending a talk on the preprint could ask. I plan to use variants of such prompts to prepare my future presentations or to begin reading a technically complex paper. Initially, I labored to make the prompts as precise as possible, based on experience with programming or scripting languages. Eventually the best results came when I unlearned that caution and simply threw lots of raw text at the AI. This level of robustness may enable AI tools to integrate with traditional software tools—or with each other, or with personal data and preferences. It will disrupt workflows everywhere in a way that the current AI tools, used in isolation, merely hint at doing.
Used conversationally, GPT-4 can serve as a compassionate listener, an enthusiastic sounding board, a creative muse, a translator or teacher, or a devil’s advocate. They could help us flourish in any number of dimensions.
Because these tools allow for a wide variety of inputs, we are still experimenting with how to use them to their full potential. I now routinely use GPT-4 to answer casual and vaguely phrased questions that I would previously have attempted with a carefully prepared search-engine query. I have asked it to suggest first drafts of complex documents I had to write. Others that I know have used the remarkable artificial emotional intelligence of these tools to obtain support, comfort, and a safe environment to explore their feelings. One of my colleagues was moved to tears by a GPT-4-generated letter of condolence to a relative who had recently received a devastating medical diagnosis. Used conversationally, GPT-4 can serve as a compassionate listener, an enthusiastic sounding board, a creative muse, a translator or teacher, or a devil’s advocate. They could help us flourish in any number of dimensions.
Current large language models (LLM) can often persuasively mimic correct expert response in a given knowledge domain (such as my own, research mathematics). But as is infamously known, the response often consists of nonsense when inspected closely. Both humans and AI need to develop skills to analyze this new type of text. The stylistic signals that I traditionally rely on to “smell out” a hopelessly incorrect math argument are of little use with LLM-generated mathematics. Only line-by-line reading can discern if there is any substance. Strangely, even nonsensical LLM-generated math often references relevant concepts. With effort, human experts can modify ideas that do not work as presented into a correct and original argument. The 2023-level AI can already generate suggestive hints and promising leads to a working mathematician and participate actively in the decision-making process. When integrated with tools such as formal proof verifiers, internet search, and symbolic math packages, I expect, say, 2026-level AI, when used properly, will be a trustworthy co-author in mathematical research, and in many other fields as well.
Then what? That depends not just on the technology, but on how existing human institutions and practices adapt. How will research journals change their publishing and referencing practices when entry-level math papers for AI-guided graduate students can now be generated in less than a day—and with the far better accuracy of future AI tools? How will our approach to graduate education change? Will we actively encourage and train our students to use these tools?
We are largely unprepared to address these questions. There will be shocking demonstrations of AI-assisted achievement and courageous experiments to incorporate them into our professional structures. But there will also be embarrassing mistakes, controversies, painful disruptions, heated debates, and hasty decisions.
Our usual technology paradigms will not serve as an adequate guide for navigating these uncharted waters. Perhaps the greatest challenge will be transitioning to a new AI-assisted world as safely, wisely, and equitably as possible.
Check out my blog to read GPT-4’s essay on human flourishing guided by my prompts.
The view, opinion, and proposal expressed in this essay is of the author and does not necessarily reflect the official policy or position of any other entity or organization, including Microsoft and OpenAI. The author is solely responsible for the accuracy and originality of the information and arguments presented in their essay. The author’s participation in the AI Anthology was voluntary and no incentives or compensation was provided.
Tao, T. (2023, June 12). Embracing Change and Resetting Expectations. In: Eric Horvitz (ed.), AI Anthology. https://unlocked.microsoft.com/ai-anthology/terence-tao
Terence Tao
Terence Tao is a professor of Mathematics at UCLA; his areas of research include harmonic analysis, PDE, combinatorics, and number theory. He has received a number of awards, including the Fields Medal in 2006. Since 2021, Tao also serves on the President’s Council of Advisors on Science and Technology.
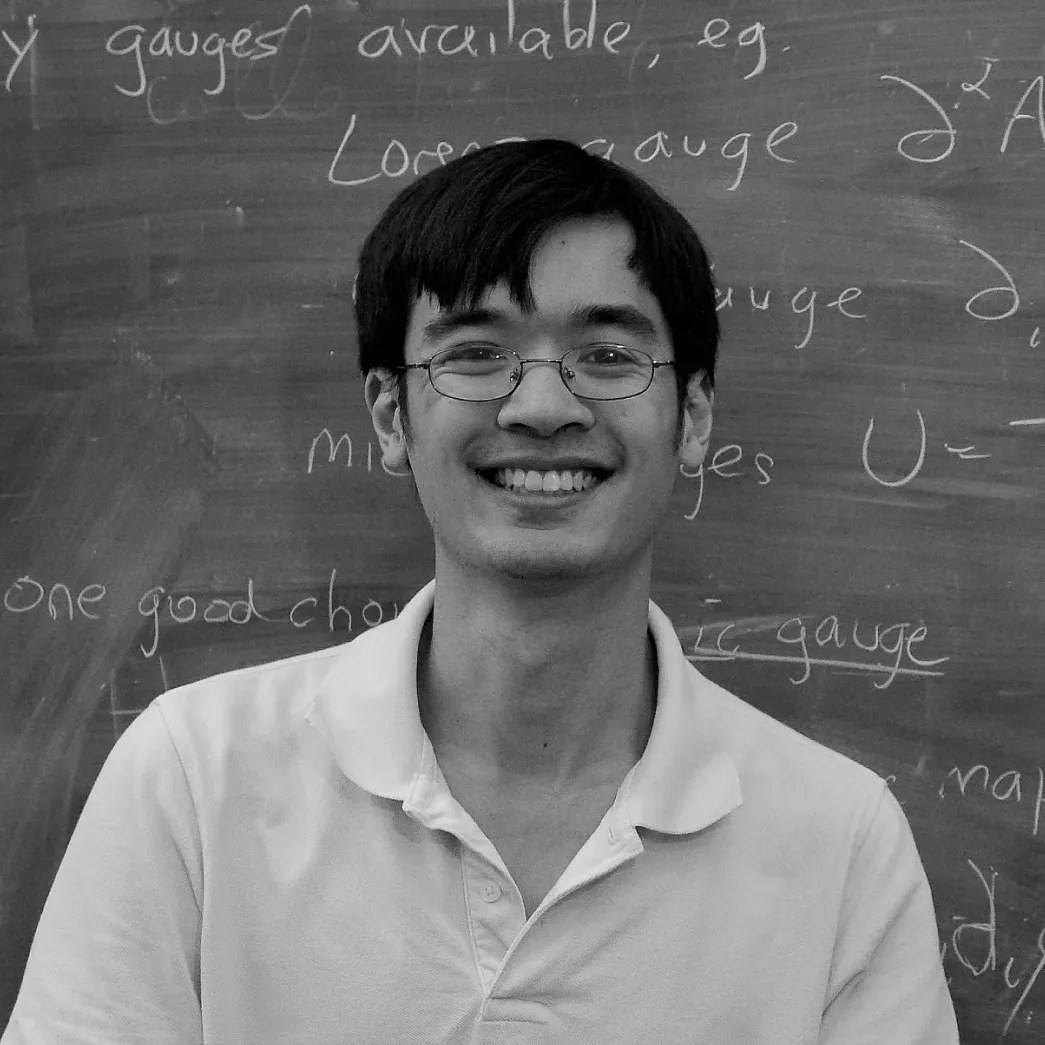